For scientists and engineers, the best way to understand a new or unknown material—whether it’s an alloy, a pharmaceutical or a meteorite—is to delve into its atoms.
Techniques such as X-ray diffraction, microscopy and spectroscopy can give insights into a material’s crystal orientation, structure and chemical composition, information that’s often vital for predicting the performance of advanced materials such as nuclear fuels.
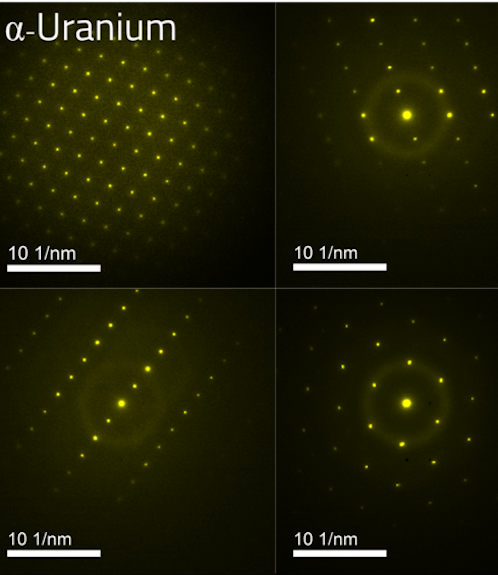
But, analyzing data from these methods, especially diffraction patterns, is a time-consuming process.
Now, Idaho National Laboratory researchers have helped develop a computer model that can interpret diffraction patterns in hours instead of weeks. The research appears in the journal Science Advances.
A diffraction pattern is the result of a beam of light, X-rays, neutrons or electrons scattering off a well-ordered or amorphous crystalline material. The crystals bend the beam into a particular pattern that is projected onto a camera sensor or photographic paper. Interpreting the patterns provides a knowledge of the underlying material structure down to the local arrangement of atoms.
Until now, interpreting those raw, experimental images was difficult, said INL staff scientist Jeff Aguiar.
“Everyone’s asking, ‘What’s the crystal structure?’ and ‘What’s the coordination of the atoms?’ It’s pretty daunting for people,” he said. “They take out modern versions of a protractor and a ruler and open the Standard X-ray Diffraction Powder Patterns handbook.”
A daunting task made easier
Even with the tools and the know-how, using the current methods to analyze diffraction patterns of complex materials can take months. To prove this point, Aguiar and his colleagues sent a challenging series of diffraction patterns to experts across the country.
“We made a Google survey and sent it out to national lab folks, university professors and graduate students and asked them what the structure is,” he said. “It took anywhere from a week to six months. The individual who was the most accurate took six months.”
The new INL model came from a desire to streamline this laborious process from weeks or months to a few hours. “It’s using the data that’s out there to push the community forward from the routine analysis that we’ve all struggled with since grad school,” Aguiar said.
Machine learning using existing information
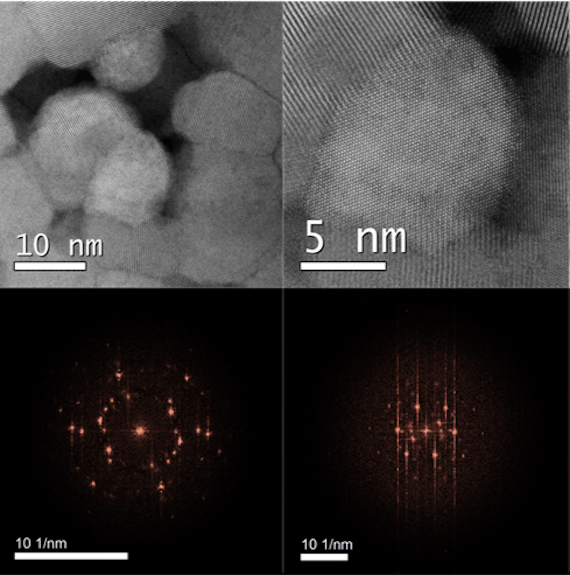
The model uses machine learning and a library of about 500,000 existing “crystal information files,” and profiles of existing crystals for the computer to use as a reference. The program turns the geometric arrangement of dots on the diffraction pattern into a 2-dimensional profile that’s easier for the model to compare and interpret. The histogram’s peaks indicate the structure of the crystal.
“It’s just leveraging all the information that’s out there, Aguiar said.
The model doesn’t give results with 100% certainty, but does gives researchers, some of whom might generate terabytes of diffraction data in a day, an important tool that can quickly suggest a solution.
Just as crucial, the model gives researchers the ability to measure crystal structures in new ways over different time scales.
In one experiment, Aguiar and his colleagues used the model to help observe the evolution of a crystal as it melted and solidified under the heat of a laser. Cameras captured a series of diffraction patterns at 10 microseconds apart, and the model was able to predict with good accuracy the crystal structure of the powder throughout, the crystal structure of the end material and when that crystal structure changed.
“If a model like this didn’t exist, you might never see these transitions in the timeline of the study,” Aguiar said.
Answering challenging questions with confidence
The researchers are now applying the same modeling techniques to imaging and spectroscopy.
As with crystal diffraction, the model compares imaging and spectroscopy data with known samples and provides researchers with probable solutions.
“If you have a diffraction dataset that is paired with imaging or spectroscopy, you can answer those really challenging questions with more confidence,” Aguiar said.
Combining different analytical methods under one model has a wide range of applications including pharmaceuticals, polymers, meteorites, irradiated fuels, pathogens and alloys.
“It could be used for forensic work,” Aguiar said. “It can detect counterfeit alloys and materials.”
It could also be used by scientific journals during the peer review process, he continued.
The model is available to the scientific community through Amazon Web Services. The project is a collaboration among INL; the University of Utah; Sandia National Laboratories; Oak Ridge National Laboratory; the University of Hawaii, Manoa; University of California, Irvine; and Integrated Dynamic Electron Solutions. INL’s Laboratory Directed Research & Development program funded the work.
“We’re trying to make that community grow by reaching out,” Aguiar said. “We’re eager to help.”